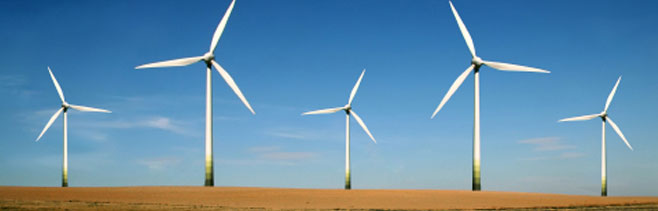
Date of Award
5-2023
Degree Type
Thesis
Degree Name
Master of Science in GIS for Development and Environment (GISDE)
Department
International Development, Community and Environment
Chief Instructor
Lyndon Estes
Keywords
cropland mapping; agriculture; U-Net; Zambia
Abstract
Cropland mapping is a crucial tool for evaluating food security. Cropland in African countries is expected to expand by at least 140M ha by 2050 to satisfy food demand. Developing accurate, large-area cropland maps of Africa’s smallholder agricultural systems is critical. To address this need, we use U-Net, a convolutional neural network, to map cropland for the year 2021 in the Republic of Zambia, a country experiencing rapid agricultural growth. To undertake this work, we first created a labeling platform and workflow protocol on Google Earth Engine (GEE) to collect labels from high spatial-resolution Planet-Scope NICFI Basemaps (4.77m) within grids of 0.005-degree resolution, resulting in labels having dimensions of 200X200 pixels. It provided the growing and off-season as well as false color composite imagery to create labels for training and evaluating the model, collecting a total of 916 over Zambia. To develop the model, we used an existing U-Net model that was trained with 5,377 labels collected across four African countries--Tanzania, Ghana, Nigeria, and the Republic of Congo. The model was trained to recognize three classes (field interior, field boundary, and non-field). This model was then adapted to Zambia by fine-tuning the last 21 layers of the decoder for 300 epochs, using 80% of the 916 collected labels for training and 20% for validation, resulting in an F1 score of 0.64 for the field interior class. The fine-tuned model was used to map predictions for the field interior class for a representative set of 0.05 degree image tiles for the entire country. The results created a 3-meter resolution crop field map Zambia. The accuracy of the map was evaluated with 100 independent reference points, showing an overall, user’s, and producer’s accuracy of 79, 64, and 91%. The study suggests future opportunities to improve the model by balancing the number of labels among different classes, incorporating slope or DEM data as additional channels to train the model, combining field interior class prediction with field boundary class prediction to reduce false negatives, and applying different CNN architectures in Zambia, such as DenseNet or ResNet.
Recommended Citation
Yao, Yao-Ting, "Country-Scale Crop Field Mapping for Zambia Using U-Net" (2023). International Development, Community and Environment (IDCE). 261.
https://commons.clarku.edu/idce_masters_papers/261