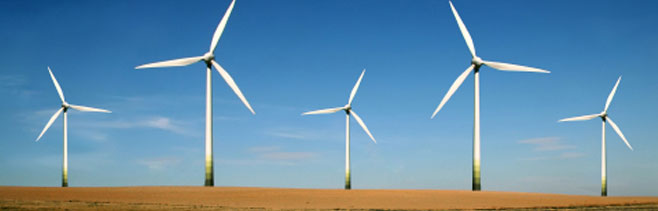
Date of Award
8-2020
Degree Type
Thesis
Degree Name
Master of Science in GIS for Development and Environment (GISDE)
Department
International Development, Community and Environment
Chief Instructor
J. Ronald Eastman, Lyndon Estes
Keywords
image times series; teleconnection; climate; Empirical Orthogonal Teleconnection; Principal Components Analysis, Empirical Orthogonal Functions, autoencoder
Abstract
The Earth system is considered to possess certain modes - preferred patterns of variability that can represent the latent structure of the climate system, also known as teleconnections. There are approaches to discover these patterns, Principal Components Analysis and Empirical Orthogonal Teleconnection (EOT) analysis. However, while the latter is very effective, it is computationally intensive. An autoencoder is an unsupervised neural network that learns an efficient neural representation of input data. It is considered as a dimensionality reduction tool that is highly similar to PCA and EOT. The hidden layer of an autoencoder represents the most significant information of the input, which can extract crucial latent structures. When applying Earth Observation data in a linear autoencoder, the information presented in the nodes is teleconnections. However, with an increased number of nodes in the hidden layer, teleconnections spread across nodes and the patterns become mixed. This research presents a sequential autoencoder (SA) with only one single node in the hidden layer based on subsequent residual series analysis. It has a major finding that the hidden nodes of SA are identical to the corresponding PCA components.
Recommended Citation
He, Jiena, "Application of Autoencoders for Latent Pattern Analysis in Image Time Series" (2020). Sustainability and Social Justice. 244.
https://commons.clarku.edu/idce_masters_papers/244
Included in
Atmospheric Sciences Commons, Climate Commons, Other Physical Sciences and Mathematics Commons