Geography
An evaluation of bagging, boosting, and random forests for land-cover classification in Cape Cod, Massachusetts, USA
Document Type
Article
Abstract
The iterative and convergent nature of ensemble learning algorithms provides potential for improving classification of complex landscapes. This study performs land-cover classification in a heterogeneous Massachusetts landscape by comparing three ensemble learning techniques (bagging, boosting, and random forests) and a non-ensemble learning algorithm (classification trees) using multiple criteria related to algorithm and training data characteristics. The ensemble learning algorithms had comparably high accuracy (Kappa range: 0.76-0.78), which was 11% higher than that of classification trees. Ensemble learning techniques were not influenced by calibration data variability, were robust to one-fifth calibration data noise, and insensitive to a 50% reduction in calibration data size.
Publication Title
GIScience and Remote Sensing
Publication Date
2012
Volume
49
Issue
5
First Page
623
Last Page
643
ISSN
1548-1603
DOI
10.2747/1548-1603.49.5.623
Keywords
accuracy assessment, algorithm, calibration, forest cover, image classification, land cover, performance assessment, remote sensing
Repository Citation
Ghimire, Bardan; Rogan, John; Galiano, Víctor; Panday, Prajjwal; and Neeti, Neeti, "An evaluation of bagging, boosting, and random forests for land-cover classification in Cape Cod, Massachusetts, USA" (2012). Geography. 659.
https://commons.clarku.edu/faculty_geography/659
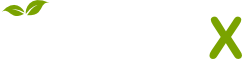
- Citations
- Citation Indexes: 198
- Policy Citations: 1
- Usage
- Abstract Views: 6
- Captures
- Readers: 207