Biology
Document Type
Article
Abstract
Background: The recent development of metagenomic sequencing makes it possible to massively sequence microbial genomes including viral genomes without the need for laboratory culture. Existing reference-based and gene homology-based methods are not efficient in identifying unknown viruses or short viral sequences from metagenomic data. Methods: Here we developed a reference-free and alignment-free machine learning method, DeepVirFinder, for identifying viral sequences in metagenomic data using deep learning. Results: Trained based on sequences from viral RefSeq discovered before May 2015, and evaluated on those discovered after that date, DeepVirFinder outperformed the state-of-the-art method VirFinder at all contig lengths, achieving AUROC 0.93, 0.95, 0.97, and 0.98 for 300, 500, 1000, and 3000 bp sequences respectively. Enlarging the training data with additional millions of purified viral sequences from metavirome samples further improved the accuracy for identifying virus groups that are under-represented. Applying DeepVirFinder to real human gut metagenomic samples, we identified 51,138 viral sequences belonging to 175 bins in patients with colorectal carcinoma (CRC). Ten bins were found associated with the cancer status, suggesting viruses may play important roles in CRC. Conclusions: Powered by deep learning and high throughput sequencing metagenomic data, DeepVirFinder significantly improved the accuracy of viral identification and will assist the study of viruses in the era of metagenomics. [Figure not available: see fulltext.]
Publication Title
Quantitative Biology
Publication Date
3-2020
Volume
8
Issue
1
First Page
64
Last Page
77
ISSN
2095-4689
DOI
10.1007/s40484-019-0187-4
Keywords
deep learning, machine learning, metagenome, virus identification
Repository Citation
Ren, Jie; Song, Kai; Deng, Chao; Ahlgren, Nathan A.; Fuhrman, Jed A.; Li, Yi; Xie, Xiaohui; Poplin, Ryan; and Sun, Fengzhu, "Identifying viruses from metagenomic data using deep learning" (2020). Biology. 58.
https://commons.clarku.edu/faculty_biology/58
Creative Commons License
This work is licensed under a Creative Commons Attribution 4.0 International License.
Copyright Conditions
Ren, J., Song, K., Deng, C., Ahlgren, N. A., Fuhrman, J. A., Li, Y., ... & Sun, F. (2020). Identifying viruses from metagenomic data using deep learning. Quantitative Biology, 8(1), 64-77 https://doi.org/10.1007/s40484-019-0187-4
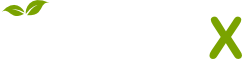
- Citations
- Citation Indexes: 383
- Usage
- Downloads: 30
- Captures
- Readers: 425
- Mentions
- Blog Mentions: 1
- News Mentions: 5
- References: 1